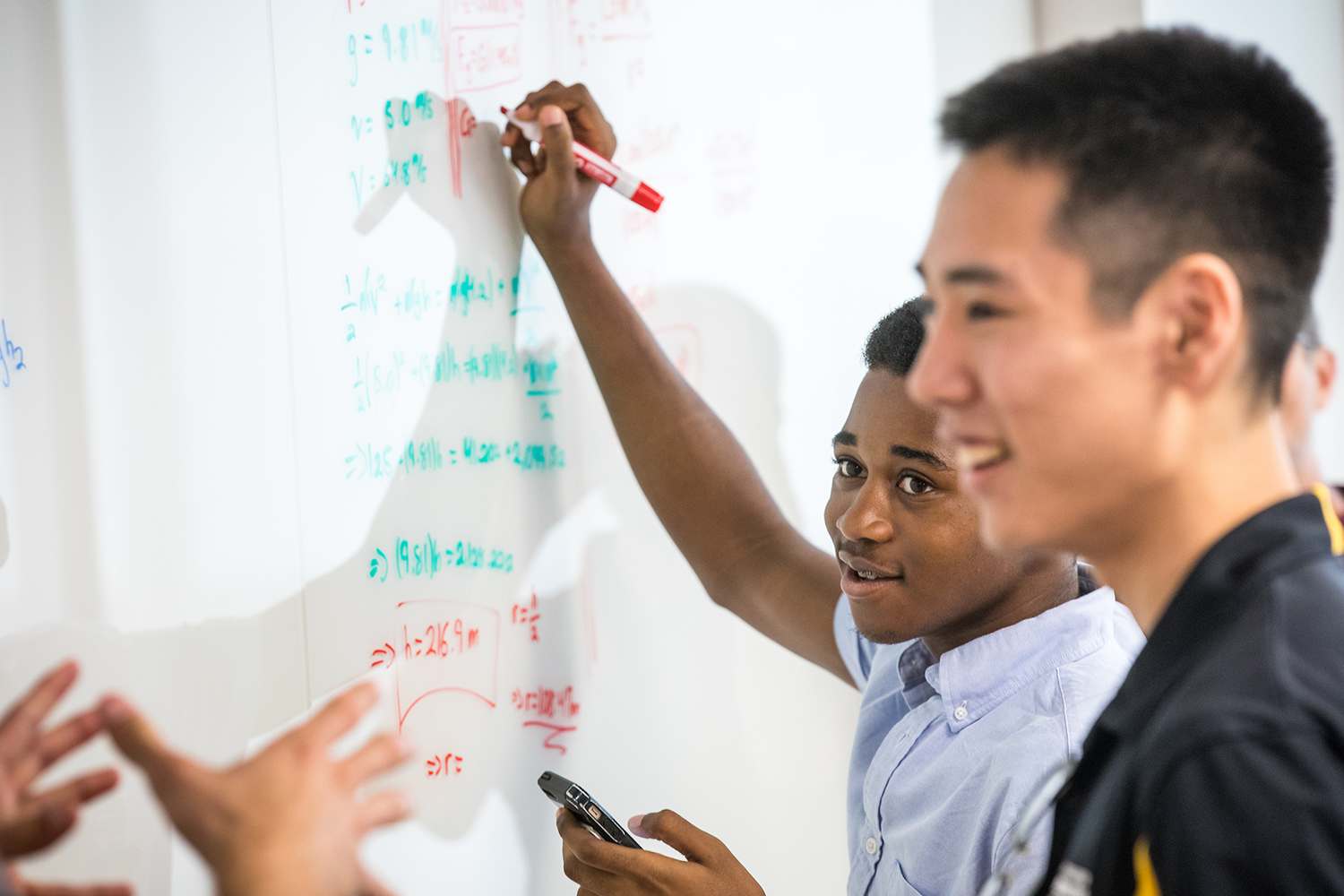
The Quantitative Methodology Group
The T. Denny Sanford School of Social and Family Dynamics (The Sanford School) houses a group of faculty members who study, develop, critique, apply, and teach quantitative methods.
Who We Are
Faculty members affiliated with The Sanford School’s Quantitative Methodology Group and their methodological interests are as follows:
Education in Quantitative Methodology
Undergraduate Student Courses
- Social Statistics I
- Multivariate Statistics for Social Sciences
Graduate Student Courses
A modernized introductory sequence and many elective courses
- Introduction to Regression and Linear Models
- Advanced Regression and Nonlinear Models
- Exploratory and Confirmatory Factor Analysis for the Social Sciences
- Structural Equation Analysis for the Social Sciences
- Structural Equation Modeling with Longitudinal Data
- Pattern Centered Analysis
- Latent Growth and Longitudinal Mixture Models
- Bayesian Analyses in the Social Sciences
- Advanced Bayesian Methods
- Multilevel, Time Varying, and Related Models
Postdoctoral Fellows
We train, and collaborate with, postdoctoral fellows who have primary interests in statistical and measurement methodologies.
- Where are they now?
- Keke Lai (postdoctoral fellow 2012-2014) - Associate Professor in Quantitative Methods, Measurement, and Statistics at UC Merced
- Yan Xia (postdoctoral fellow 2016-2018) - Assistant Professor in Educational Psychology at the University of Illinois Urbana-Champaign
- Yixing Liu (postdoctoral fellow 2019-2020) - Associate Professor in the School of Management at Beijing University of Chinese Medicine
Workshops for Faculty and Students
Previous Events
Sample-Size Planning (Keke Lai) - January 2023
Missing Data Analysis (Craig Enders) – January 2020
Bayesian Modeling (Roy Levy) – January 2020
Lunch-and-Learn Meetings
Our faculty members, postdoctoral fellows, and graduate students participate in lunch-and-learn meetings focused on teaching an analytic strategy, discussing issues in the field, or presenting new methodological research.
Research in Quantitative Methodology
Faculty members in the Quantitative Methodology Group vary in their emphasis on applied and methodological research. We serve as a resource for The Sanford School and ASU more broadly though research collaborations with faculty members and students. We participate in group and in individual research activities.
Levy, R., & McNeish, D. (2021). Perspectives on Bayesian inference and their implications for data analysis. Psychological Methods. https://doi.org/10.1037/met0000443
Levy, R. (2019). Dynamic Bayesian network modeling of game-based diagnostic assessments. Multivariate Behavioral Research, 54, 771-794. https://doi.org/10.1080/00273171.2019.1590794
Levy, R. (2017). Distinguishing outcomes from indicators via Bayesian modeling. Psychological Methods, 22, 632-648. https://doi.org/10.1037/met0000114
Savord, A., McNeish, D., Iida, M., Quiroz, S., & Ha, T. (in press). Fitting the Longitudinal Actor-Partner Interdependence Model as a Dynamic Structural Equation Model in Mplus. Structural Equation Modeling: A Multidisciplinary Journal.
Iida, M., Seidman, G., & Shrout, P. E. (2018). Models of interdependent individuals and dyadic process in relationship research. Journal of Social and Personal Relationship, 35(1), 59-88. https://doi.org/10.1177/0265407517725407
Iida, M., Shrout, P. E., Laurenceau, J. P., & Bolger, N. (in press). Using intensive longitudinal methods in psychological research. In H. Cooper, P.M. Camic, D. L. Long, A. T., Panter, D. Rindskopf & K. J. Sher (Eds.), APA Handbook of Research Methods in Psychology, 2nd Ed., Vol. 1: Foundations, Planning, Measures and Psychometrics. Washington, DC: American Psychological Association.
Hinnant, B., Schulenberg, J., Jager, J. (2021). Multifinality, equifinality, and fanning: Developmental concepts and statistical implications. International Journal of Behavioral Development, 45(5), 429-439. https://doi.org/10.1177/01650254211020402
Levy, R. (2020). Implications of considering response process data for greater and lesser psychometrics. Educational Assessment, 25, 218-235. https://doi.org/10.1080/10627197.2020.1804352
Li, L., Sheehan, C. M., & Thompson, M. S. (2019). Measurement invariance and sleep quality differences between men and women in the Pittsburgh Sleep Quality Index. Journal of Clinical Sleep Medicine, 15(12), 1769-1776. https://doi.org/10.5664/jcsm.8082
Sheehan, C. M., & Tucker-Drob, E. M. (2019). Gendered expectations distort male–female differences in instrumental activities of daily living in later adulthood. The Journals of Gerontology: Series B, 74(4), 715-723. http://www.doi.org/10.1093/geronb/gbw209
Fay, D. M., Levy, R., & Mehta, V. (2018). Investigating psychometric isomorphism for traditional and performance-based assessment. Journal of Educational Measurement, 55, 52-77. https://www.doi.org/10.1111/jedm.12163
Sheehan, C., Powers, D., Margerison-Zilko, C., McDevitt, T., & Cubbin, C. (2018). Historical neighborhood poverty trajectories and child sleep. Sleep Health, 4(2), 127-134. https://www.doi.org/10.1016/j.sleh.2017.12.005
DiCerbo, K. E., Xu, Y., Levy, R., Lai, E., & Holland, L. (2017). Modeling student cognition in digital and nondigital assessment environments. Educational Assessment, 22, 275-297. https://www.doi.org/10.1080/10627197.2017.1382343
DeLay, D. & Bukowski, W. M. (2021). Multilevel models and multidisciplinary perspectives: Bringing peer relations research into the future. Merrill-Palmer Quarterly, 67(4), 509-524.
Jager, J., Putnick, D. L., & Bornstein, M. H. (2017). More than just convenient: The scientific merits of homogeneous convenience samples. In N. A. Card (Ed.), Developmental Methodology. Monographs of the Society for Research in Child Development, 82(2), 13-30. https://doi.org/10.1111/mono.12296
Davis-Kean, P. E., & Jager, J. (2017). From small to big: Methods for incorporating large-scale data into Developmental Science. In N. A. Card (Ed.), Developmental Methodology. Monographs of the Society for Research in Child Development, 82(2), 31-45. https://doi.org/10.1111/mono.12297
DeLay, D., Laursen, B., Kiuru, N., Rogers, A., & Kindermann, T. (2021). A comparison of dyadic and social network assessments of peer influence. International Journal of Behavioral Development, 45, 275-288. https://doi.org/10.1177/0165025421992866
Thompson, M. S., & Liu, Y. (in press). Flexible SEM approaches for analyzing means. In R. H. Hoyle (Ed.), Handbook of Structural Equation Modeling, 2nd ed. (pp. 389-412). New York: Guilford Press.
Gray, S., Levy, R., Alt, M., Hogan, T. P., & Cowan, N. (2022). Working memory predicts new word learning over and above existing vocabulary and nonverbal IQ. Journal of Speech, Language, and Hearing Research, 65, 1044-1069. https://doi.org/10.1044/2021_JSLHR-21-00397
Liu, Y., & Thompson, M. S. (2022). The impact of partial measurement invariance on between-group comparisons of second-order factor means. Structural Equation Modeling, 29, 86-100. https://doi.org/10.1080/10705511.2021.1936535
Liu, Y., & Thompson, M. S. (2022). The impact of DIF on general factor mean difference estimation for bifactor ordinal data. Journal of Experimental Education, 90, 981-1002. http://dx.doi.org/10.1080/00220973.2021.1926895
Liu, Y., & Thompson, M. S. (2021). General factor mean difference estimation in bifactor models with ordinal data. Structural Equation Modeling, 28, 423-439. https://doi.org/10.1080/10705511.2020.1833732
Lai, K., Green, S. B., Levy, R., Reichenberg, R., Xu, Y., Thompson, M. S., Yel, N., Eggum-Wilkens, N. D., Kunze, K. L., & Iida, M. (2016). Assessing model similarity in structural equation modeling. Structural Equation Modeling: An Multidisciplinary Journal, 23(4), 491-506. https://doi.org/10.1080/10705511.2016.1154464
Maslowsky, J., Jager, J., & Hemken, D. (2015). Interpreting latent variable interactions: Applications and extensions of the latent moderated structural equations method. International Journal of Behavioral Development, 39(1), 87-96. https://doi.org/10.1177/0165025414552301
For additional information, please contact:
Dr. Natalie Eggum, Coordinator for the Quantitative Methodology Group
Email: [email protected]
(480) 727-6899